【W(wǎng)SN】異構(gòu)無線傳感器網(wǎng)絡(luò)的開發(fā)分布式節(jié)能集群算法(DDEEC)附matlab代碼
?作者簡介:熱愛科研的Matlab仿真開發(fā)者,修心和技術(shù)同步精進(jìn),matlab項目合作可私信。
??個人主頁:Matlab科研工作室
??個人信條:格物致知。
更多Matlab完整代碼及仿真定制內(nèi)容點(diǎn)擊??
智能優(yōu)化算法 ? ? ? 神經(jīng)網(wǎng)絡(luò)預(yù)測 ? ? ? 雷達(dá)通信 ? ? ?無線傳感器 ? ? ? ?電力系統(tǒng)
信號處理 ? ? ? ? ? ? ?圖像處理 ? ? ? ? ? ? ? 路徑規(guī)劃 ? ? ? 元胞自動機(jī) ? ? ? ?無人機(jī)
?? 內(nèi)容介紹
無線傳感器網(wǎng)絡(luò)(Wireless Sensor Network,簡稱WSN)是一種由大量分布式傳感器節(jié)點(diǎn)組成的網(wǎng)絡(luò),用于監(jiān)測和收集環(huán)境中的信息。WSN的應(yīng)用范圍廣泛,包括環(huán)境監(jiān)測、農(nóng)業(yè)、醫(yī)療、交通等領(lǐng)域。然而,WSN面臨著能源有限、節(jié)點(diǎn)功耗不平衡等問題,這些問題嚴(yán)重影響了網(wǎng)絡(luò)的性能和壽命。
為了解決WSN中的能源問題,研究人員提出了各種能源節(jié)約的算法和協(xié)議。其中,分布式節(jié)能集群算法(Distributed Energy-Efficient Clustering,簡稱DDEEC)是一種常用的算法。DDEEC算法通過將網(wǎng)絡(luò)節(jié)點(diǎn)劃分為多個集群,每個集群由一個簿記節(jié)點(diǎn)(Cluster Head)負(fù)責(zé)管理和協(xié)調(diào)。簿記節(jié)點(diǎn)負(fù)責(zé)收集并匯總集群內(nèi)其他節(jié)點(diǎn)的數(shù)據(jù),并將數(shù)據(jù)傳輸?shù)交尽?/p>
DDEEC算法的核心思想是通過選擇合適的簿記節(jié)點(diǎn)來減少網(wǎng)絡(luò)中節(jié)點(diǎn)的能量消耗。在算法的初始階段,每個節(jié)點(diǎn)都有機(jī)會成為簿記節(jié)點(diǎn)。節(jié)點(diǎn)根據(jù)其剩余能量和與基站的距離來計算能量消耗指標(biāo),選擇能量消耗最小的節(jié)點(diǎn)作為簿記節(jié)點(diǎn)。選定簿記節(jié)點(diǎn)后,其他節(jié)點(diǎn)將加入到與其距離最近的簿記節(jié)點(diǎn)所在的集群中。
為了進(jìn)一步減少能量消耗,DDEEC算法引入了輪流工作的概念。每個集群中的節(jié)點(diǎn)將根據(jù)輪流工作的規(guī)則,在不同時間段內(nèi)交替充當(dāng)簿記節(jié)點(diǎn)和普通節(jié)點(diǎn)。這樣可以平衡網(wǎng)絡(luò)中節(jié)點(diǎn)的能量消耗,延長整個網(wǎng)絡(luò)的壽命。
DDEEC算法在實際應(yīng)用中取得了良好的效果。通過合理地選擇簿記節(jié)點(diǎn),能夠減少節(jié)點(diǎn)之間的通信開銷,提高網(wǎng)絡(luò)的能量利用率。同時,通過輪流工作的機(jī)制,能夠避免節(jié)點(diǎn)能量消耗不均衡的問題,延長整個網(wǎng)絡(luò)的壽命。
然而,DDEEC算法也存在一些挑戰(zhàn)和限制。首先,算法需要節(jié)點(diǎn)之間進(jìn)行頻繁的通信,增加了網(wǎng)絡(luò)的能量消耗。其次,算法在網(wǎng)絡(luò)規(guī)模較大時,簿記節(jié)點(diǎn)的選擇可能會變得復(fù)雜和困難。此外,算法對網(wǎng)絡(luò)拓?fù)浣Y(jié)構(gòu)的依賴較強(qiáng),不適用于某些特殊環(huán)境下的應(yīng)用。
為了進(jìn)一步提高WSN的能源利用效率,研究人員需要繼續(xù)改進(jìn)和優(yōu)化DDEEC算法,解決其存在的問題和限制。同時,還需要開展更多的實驗和仿真研究,驗證算法的性能和可行性。
總之,DDEEC算法是一種有效的分布式節(jié)能集群算法,可以在異構(gòu)無線傳感器網(wǎng)絡(luò)中減少節(jié)點(diǎn)的能量消耗,延長網(wǎng)絡(luò)的壽命。然而,該算法仍然存在一些挑戰(zhàn)和限制,需要進(jìn)一步的研究和改進(jìn)。通過持續(xù)的努力,相信可以開發(fā)出更加高效和可靠的節(jié)能算法,推動WSN技術(shù)的發(fā)展和應(yīng)用。
?? 部分代碼
clear all
xm=100;
ym=100;
sink.x=0.5*xm; ?%location of sink on x-axis
sink.y=0.5*ym; ?%location of sink on y-axis
n=100 ?%nodes
P=0.1; ?%probability of cluster heads
Eo=0.5;%initial energy
%
Echeck=Eo;
%
ETX=50*0.000000001; ?%tx energy
ERX=50*0.000000001; ?%rx energy
Efs=10*0.000000000001; ?%free space loss
Emp=0.0013*0.000000000001; ? %multipath loss
%Data Aggregation Energy
EDA=5*0.000000001; ?%compression energy
a=1; ? %fraction of energy enhancment of advance nodes
rmax=5000 ?%maximum number of rounds
do=sqrt(Efs/Emp); ?%distance do is measured
Et=0; ?%variable just use below
m=0;
mony=0;
c=0.02;
b=0.7;
talhar=0;
for i=1:1:n
? ?S(i).xd=rand(1,1)*xm; ?%generates a random no. use to randomly distibutes nodes on x axis
? ?XR(i)=S(i).xd;
? ?S(i).yd=rand(1,1)*ym; ?%generates a random no. use to randomly distibutes nodes on y axis
? ?YR(i)=S(i).yd;
? ?S(i).G=0; %node is elegible to become cluster head
? ?talhar=rand*a
? ?S(i).E=Eo*(1+talhar);
? ?S(i).A=talhar;
? ?E(i)= S(i).E;
? ?if (E(i)>Echeck)
? ? ? ?mony=mony+1;
? ?end
? ?Et=Et+E(i); ?%estimating total energy of the network
? ?%initially there are no cluster heads only nodes
? ?S(i).type='N';
end
m=mony/100;
d1=0.765*xm/2; ?%distance between cluster head and base station
K=sqrt(0.5*n*do/pi)*xm/d1^2; %optimal no. of cluster heads
d2=xm/sqrt(2*pi*K); ?%distance between cluster members and cluster head
Er=4000*(2*n*ETX+n*EDA+K*Emp*d1^4+n*Efs*d2^2); ?%energy desipated in a round
S(n+1).xd=sink.x; %sink is a n+1 node, x-axis postion of a node
S(n+1).yd=sink.y; %sink is a n+1 node, y-axis postion of a node
countCHs=0; ?%variable, counts the cluster head
cluster=1; ?%cluster is initialized as 1
flag_first_dead=0; %flag tells the first node dead
flag_teenth_dead=0; ?%flag tells the 10th node dead
flag_all_dead=0; ?%flag tells all nodes dead
?? 運(yùn)行結(jié)果
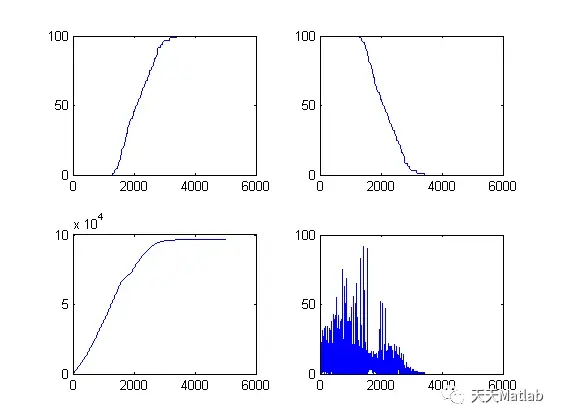