【直播預(yù)告】SFFAI 136 通信強(qiáng)化學(xué)習(xí)專題
SFFAI論壇已開放注冊
詳情點(diǎn)擊查看:https://bbs.sffai.com/d/312
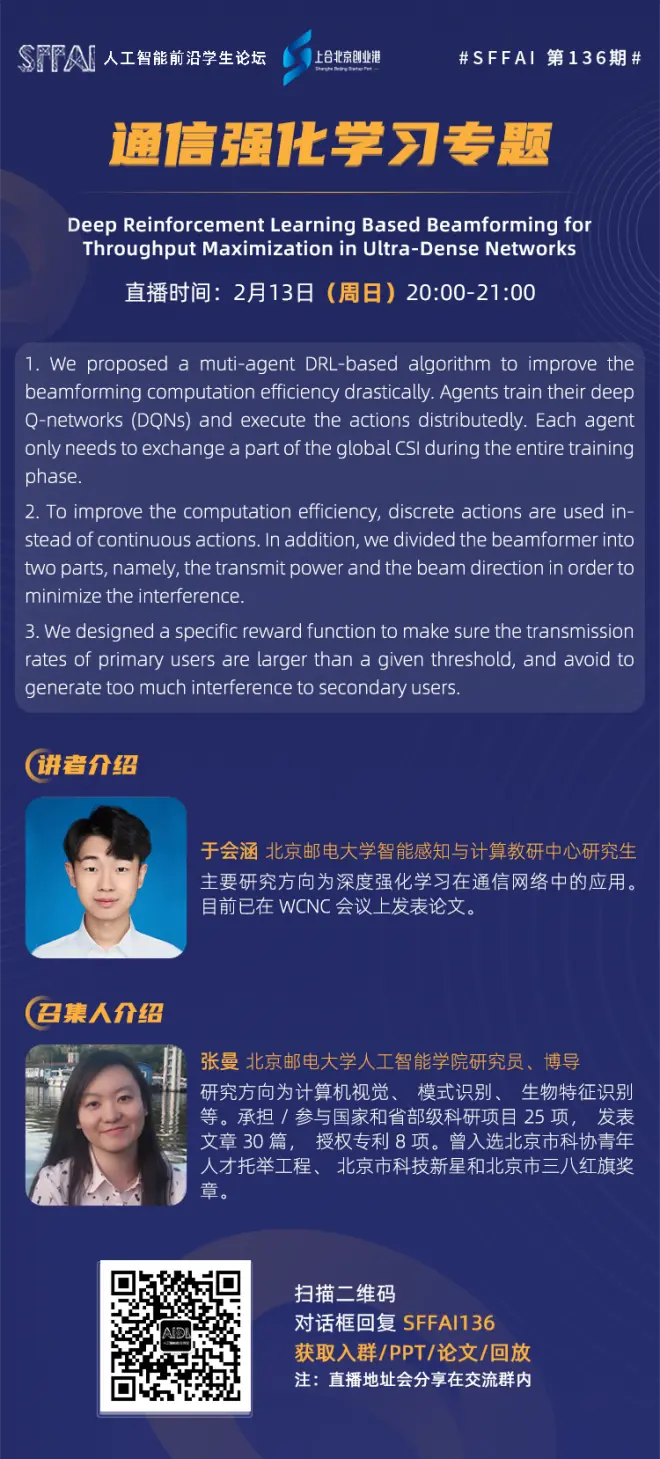
講者介紹
于會涵,北京郵電大學(xué)智能感知與計算教研中心研究生,主要研究方向?yàn)樯疃葟?qiáng)化學(xué)習(xí)在通信網(wǎng)絡(luò)中的應(yīng)用。目前已在WCNC會議上發(fā)表論文。
分享題目
Deep Reinforcement Learning Based Beamforming for Throughput Maximization in Ultra-Dense Networks
分享摘要
Ultra-dense network (UDN) is a promising technology for 5G and beyond communication systems to meet the requirements of explosive data traffic. However, the dense distribution of wireless terminals potentially leads to severe interference and deteriorate network performance. To address this issue, beamforming is widely used to coordinate the interference in UDNs and improve receive gains by controlling the phase of multiple antennas. In this paper, we propose a multi-agent deep reinforcement learning (DRL) based beamforming algorithm to achieve more dynamic and fast beamforming adjustment. In the proposed algorithm, the agents inside beamforming controllers are distributively trained while exchanging partial channel state information (CSI) for better optimizing beamforming vectors to achieve maximized throughputs in UDNs. The evaluation results demonstrate that the proposed algorithm significantly improves the computation efficiency, as well as achieves the highest network throughput compared to several baselines.
分享亮點(diǎn)
1. We proposed a muti-agent DRL-based algorithm to improve the beamforming computation efficiency drastically. Agents train their deep Q-networks (DQNs) and execute the actions distributedly. Each agent only needs to exchange a part of the global CSI during the entire training phase.
2.?To improve the computation efficiency, discrete actions are used instead of continuous actions. In addition, we divided the beamformer into two parts, namely, the transmit power and the beam direction in order to minimize the interference.
3.?We designed a specific reward function to make sure the transmission rates of primary users are larger than a given threshold, and avoid to generate too much interference to secondary users.?
直播時間
2022年2月13日(周日)20:00—21:00 線上直播
關(guān)注微信公眾號:人工智能前沿講習(xí),對話框回復(fù)“SFFAI136”,獲取入群二維碼
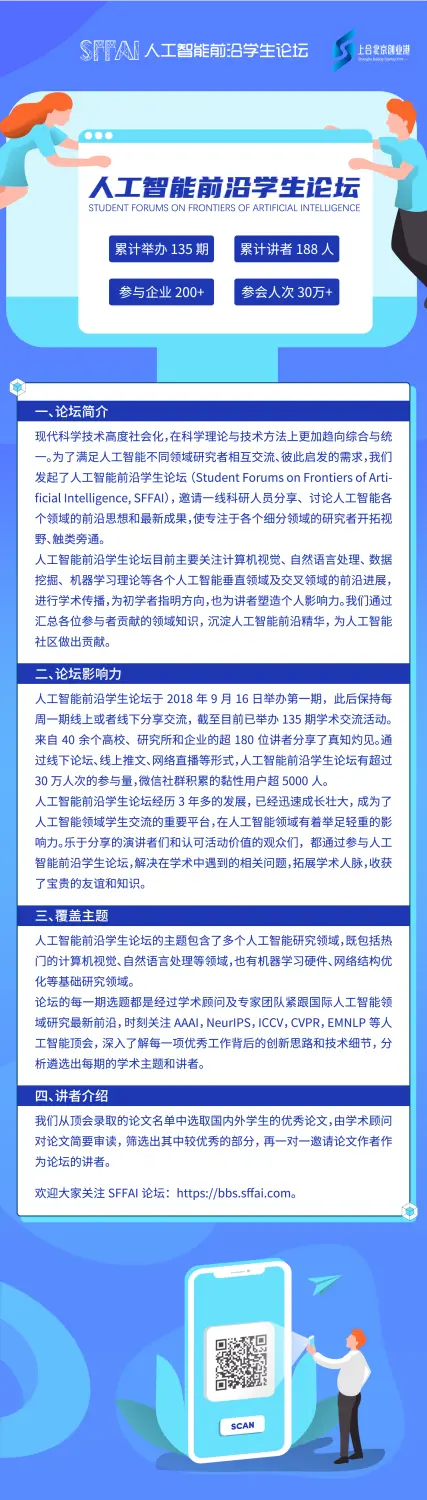