什么是數(shù)據(jù)科學(xué)?《What is data science》 by Mike Loukides翻譯和精讀01
(根據(jù)百度學(xué)術(shù)顯示,Mike Loukides在2010年于《Oreilley Media》發(fā)表的報(bào)告《What is data science》一共有94次引用,這是對(duì)這篇報(bào)告的翻譯和精讀)
What is data science?數(shù)學(xué)科學(xué)是什么?
Mike Loukides 多倫多公共實(shí)驗(yàn)室的Mike Loukides
?
Table of Contents ?目錄
What is data science? . . . . . . . . . . . . . . . . . . . . . . . . . . . . . . . . . . . . . .1
什么是數(shù)據(jù)科學(xué)?. . . . . . . . . . . . . . . . . . . . . . . . . . . . . . . . . . . . . . . .1
The future belongs to the companies and people that turn data into products ???????????????????????????????????????????????1
未來(lái)屬于能將數(shù)據(jù)轉(zhuǎn)化為產(chǎn)品的公司和人 ??????????????????1
What is data science? ?????????????????????????????????????1
什么是數(shù)據(jù)科學(xué)? ??????????????????????????????????????1
Where data comes from ???????????????????????????????????4
數(shù)據(jù)從哪里來(lái) ??????????????????????????????????????????4
Working with data at scale ?????????????????????????????????7
處理大規(guī)模數(shù)據(jù) ????????????????????????????????????????7
注:at scale除了按比例,還有大規(guī)模的意思?
Making data tell its story ??????????????????????????????????10
讓數(shù)據(jù)說(shuō)出它的故事 ????????????????????????????????????10
Data scientists ??????????????????????????????????????????11
數(shù)據(jù)科學(xué)家 ????????????????????????????????????????????11
?
What is data science?
什么是數(shù)據(jù)科學(xué)?
The future belongs to the companies and people that
turn data into products
未來(lái)屬于能將數(shù)據(jù)轉(zhuǎn)化為產(chǎn)品的公司和人
We’ve all heard it: according to Hal Varian, statistics is the next sexy job. Five
years ago, in What is Web 2.0, Tim O’Reilly said that “data is the next Intel
Inside.” But what does that statement mean? Why do we suddenly care about
statistics and about data?
翻譯:依照Hal Varian的《statistics is the next sexy job》,我們都聽(tīng)到了它(指未來(lái)屬于能將數(shù)據(jù)轉(zhuǎn)化為產(chǎn)品的公司和人)。5年前(這篇報(bào)告作于2010年,這里的5年前,是2005年),在《What is Web 2.0》中,Tim O’Reilly(O’Reilly出版社創(chuàng)始人)稱“數(shù)據(jù)是下一個(gè)英特爾”。但是那句陳述意為著什么?為什么我們突然間關(guān)心統(tǒng)計(jì)學(xué)和數(shù)據(jù)?
?
解析:Intel Inside是英特爾處理器公司的標(biāo)志,電腦上有Intel Inside標(biāo)志是指內(nèi)含英特爾處理器。
?
In this post, I examine the many sides of data science -- the technologies, the
companies and the unique skill sets.
在這份報(bào)告中,我仔細(xì)地調(diào)查了數(shù)據(jù)科學(xué)的很多方面——技術(shù),公司和獨(dú)特的技巧集合。
?
What is data science?
什么是數(shù)據(jù)科學(xué)
The web is full of “data-driven apps.” Almost any e-commerce application is a data-driven application. There’s a database behind a web front end, and middleware that talks to a number of other databases and data services (credit card processing companies, banks, and so on). But merely using data isn’t
really what we mean by “data science.” A data application acquires its value from the data itself, and creates more data as a result. It’s not just an application with data; it’s a data product. Data science enables the creation of data products.
網(wǎng)上充滿了“數(shù)據(jù)驅(qū)動(dòng)的應(yīng)用”。幾乎任何電子商務(wù)應(yīng)用都是一個(gè)數(shù)據(jù)驅(qū)動(dòng)的應(yīng)用。網(wǎng)絡(luò)前端的終點(diǎn)有一個(gè)數(shù)據(jù)庫(kù),還有與一些其它數(shù)據(jù)庫(kù)和數(shù)據(jù)設(shè)備(信用卡處理公司,銀行,等等)通信的中間件。但是僅僅使用數(shù)據(jù)并不是我們說(shuō)的“數(shù)據(jù)科學(xué)”。一個(gè)數(shù)據(jù)應(yīng)用從數(shù)據(jù)自身得到價(jià)值,并因此創(chuàng)造更多數(shù)據(jù)。它(指前面提到的數(shù)據(jù)應(yīng)用)不僅僅是有著數(shù)據(jù)的應(yīng)用,它也是一個(gè)數(shù)據(jù)產(chǎn)品。數(shù)據(jù)科學(xué)使數(shù)據(jù)產(chǎn)品的創(chuàng)造變得可行。
?
解析:a number of 表示不確定,可能是不多,也可能是很多。信用卡處理,一般指信用卡的支付。But merely using data isn’t really what we mean by “data science.” what從句中,what既是is系動(dòng)詞的表語(yǔ),同時(shí)也是mean的賓語(yǔ)。acquire是及物動(dòng)詞,vt.(通過(guò)努力、能力、行為表現(xiàn))獲得;得到;購(gòu)得。as a result因此。
?
One of the earlier data products on the Web was the CDDB database. The developers of CDDB realized that any CD had a unique signature, based on the exact length (in samples) of each track on the CD. Gracenote built a database of track lengths, and coupled it to a database of album metadata (track
titles, artists, album titles). If you’ve ever used iTunes to rip a CD, you’ve taken advantage of this database. Before it does anything else, iTunes reads the length of every track, sends it to CDDB, and gets back the track titles. If you have a CD that’s not in the database (including a CD you’ve made yourself), you can create an entry for an unknown album. While this sounds simple enough, it’s
revolutionary: CDDB views music as data, not as audio, and creates new value in doing so. Their business is fundamentally different from selling music, sharing music, or analyzing musical tastes (though these can also be “data products”). CDDB arises entirely from viewing a musical problem as a data problem.
網(wǎng)上早期的數(shù)據(jù)產(chǎn)品之一是CDDB數(shù)據(jù)庫(kù)。CDDB的開(kāi)發(fā)者基于CD上每一條音軌的具體長(zhǎng)度(在樣本中),意識(shí)到任一CD有一個(gè)獨(dú)一無(wú)二的簽名。Gracenote建立了一個(gè)音軌長(zhǎng)度的數(shù)據(jù)庫(kù),并將它連接到一個(gè)專輯元數(shù)據(jù)(音軌名稱,藝術(shù)家,專輯名稱)數(shù)據(jù)庫(kù)。如果你曾經(jīng)使用iTunes來(lái)翻錄CD,你已經(jīng)抓住了這個(gè)數(shù)據(jù)庫(kù)的優(yōu)勢(shì),將它寄給CDDB,把音軌標(biāo)題拿回來(lái)。如果你有一個(gè)不在數(shù)據(jù)中的CD(包括你自己制作的CD),你可以為一個(gè)未知專輯創(chuàng)造一個(gè)條目。盡管這聽(tīng)起來(lái)很簡(jiǎn)單,它是革命性的,CDDB將音樂(lè)視為數(shù)據(jù),而不是音頻,并在這么做的同時(shí)創(chuàng)造了新的價(jià)值。他們的業(yè)務(wù)與銷(xiāo)售音樂(lè),分享音樂(lè)或分析音樂(lè)品味(盡管這些也可以成為“數(shù)據(jù)產(chǎn)品”)有根本性不同。通過(guò)將音樂(lè)性問(wèn)題視為數(shù)據(jù)問(wèn)題,CDDB出現(xiàn)了。
?
解析:couple 作動(dòng)詞是連接起來(lái),結(jié)合起來(lái)的意思。
?
Google is a master at creating data products. Here’s a few examples:
谷歌是創(chuàng)造數(shù)據(jù)產(chǎn)品的大師。這是一些例子:
?
? Google’s breakthrough was realizing that a search engine could use input other than the text on the page. Google’s PageRank?algorithm was among the first to use data outside of the page itself, in particular, the number of links pointing to a page. Tracking links made Google searches much more
useful, and PageRank has been a key ingredient to the company’s success.
谷歌的突破是意識(shí)到一個(gè)搜索引擎能夠利用輸入而不只是利用網(wǎng)頁(yè)的文本。谷歌的網(wǎng)頁(yè)排名算法最先使用網(wǎng)頁(yè)之外的數(shù)據(jù),尤其是,指向網(wǎng)頁(yè)的鏈接的數(shù)目。追蹤鏈接讓谷歌搜索更加好用,而網(wǎng)頁(yè)排名是該公司成功的關(guān)鍵要素。
?
解析:other than除了。。。,不同于的意思。谷歌的網(wǎng)頁(yè)排名,是通過(guò)哪些鏈接被更多人點(diǎn)擊,提高推薦度。
?
? Spell checking isn’t a terribly difficult problem, but by suggesting corrections to misspelled searches, and observing what the user clicks in response, Google made it much more accurate. They’ve built a dictionary of common misspellings, their corrections, and the contexts in which they occur.
拼寫(xiě)檢查不是一個(gè)可怕難題,但是通過(guò)對(duì)拼寫(xiě)錯(cuò)誤的搜索者的提示更正,并觀察用戶點(diǎn)擊回應(yīng)的內(nèi)容,谷歌讓自己更加精確。他們已經(jīng)建立了一個(gè)常用拼寫(xiě)錯(cuò)誤的字典,他們的改正和這些錯(cuò)誤發(fā)生的上下文情景。
?
注:所以不奇怪谷歌在2017年發(fā)表論文《Attention is all you need》,提出了史詩(shī)級(jí)別的transformer架構(gòu)。谷歌在做自然語(yǔ)言處理有天然優(yōu)勢(shì),和多年的深耕布局。
?
? Speech recognition has always been a hard problem, and it remains difficult. But Google has made huge strides by using the voice data they’ve collected, and has been able to integrate voice search?into their core search engine.
語(yǔ)音識(shí)別一直都是一個(gè)困難的難題,現(xiàn)在也很困難(這是2010年的文章,當(dāng)年語(yǔ)音識(shí)別還未有現(xiàn)在的突破)。但是谷歌通過(guò)使用他們收集到的語(yǔ)音邁出巨大的步伐,并且已經(jīng)能夠?qū)⒄Z(yǔ)音搜索融入到他們核心的搜索引擎中。
?
? During the Swine Flu epidemic of 2009, Google was able to track the progress of the epidemic by following searches for flu-related topics.
在2009年豬流感爆發(fā)期間,谷歌通過(guò)關(guān)注流感相關(guān)話題來(lái)追蹤流行病的過(guò)程。
注:社交網(wǎng)絡(luò)的follow是關(guān)注的意思。
?
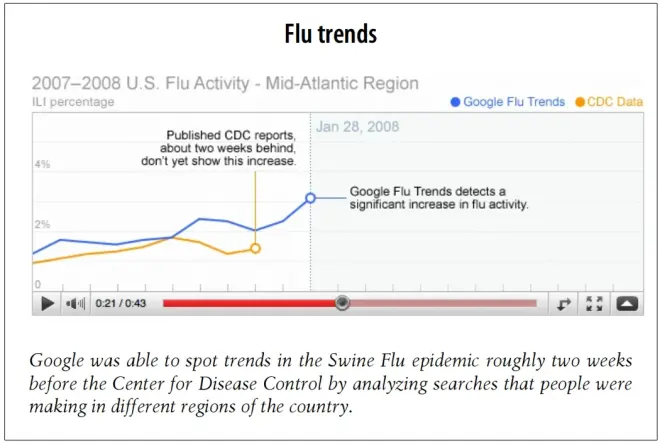
流感趨勢(shì)
2007-2008美國(guó)流感活躍度——亞特蘭大中部地區(qū)。
ILI percentage(流感樣例占門(mén)診量百分比) ?(藍(lán)色)谷歌流感趨勢(shì) ?(黃色)CDC數(shù)據(jù)
?
公布的CDC報(bào)告,關(guān)于兩周后(意思是兩周后的豎虛線時(shí)間節(jié)點(diǎn)2008年1月28日),并沒(méi)有顯示這種增長(zhǎng)。 ?
?
谷歌流感趨勢(shì)發(fā)現(xiàn)了流感活躍度的一個(gè)顯著增長(zhǎng)。
?
通過(guò)分析國(guó)家的不同地區(qū)的人們做出的搜索,谷歌有能力發(fā)現(xiàn)豬流感流行病的趨勢(shì),大約比CDC(美國(guó)中央疾控中心)早兩周時(shí)間。
?
Google isn’t the only company that knows how to use data. Facebook?and LinkedIn?use patterns of friendship relationships to suggest other people you may know, or should know, with sometimes frightening accuracy. Amazon saves your searches, correlates what you search for with what other users search for, and uses it to create surprisingly appropriate recommendations. These?recommendations are “data products” that help to drive Amazon’s more traditional retail business. They come about because Amazon understands that a book isn’t just a book, a camera isn’t just a camera, and a customer isn’t just a customer; customers generate a trail of “data exhaust” that can be mined and put to use, and a camera is a cloud of data that can be correlated with the
customers’ behavior, the data they leave every time they visit the site.
谷歌不是唯一一家知道如何使用數(shù)據(jù)的公司。臉書(shū)和LinkedIn 使用友誼關(guān)系的模式來(lái)推薦你可能認(rèn)識(shí)的其它人,或應(yīng)該認(rèn)識(shí)的,有著令人驚恐的準(zhǔn)確性。亞馬遜保存你的搜索,將你搜索的與其它用戶搜索的進(jìn)行關(guān)聯(lián),并用它創(chuàng)造令人驚訝地恰當(dāng)推薦。這些推薦就是幫助驅(qū)動(dòng)亞馬遜更多傳統(tǒng)零售商務(wù)的“數(shù)據(jù)產(chǎn)品”。它們出現(xiàn)是因?yàn)閬嗰R遜懂得一本書(shū)不止是一本書(shū),一架相機(jī)不止是一架相機(jī),一個(gè)顧客不止是一個(gè)顧客;顧客產(chǎn)生了一條可以被挖掘和投入使用的“數(shù)據(jù)排放”鏈,并且一架相機(jī)是一個(gè)可以關(guān)聯(lián)用戶行為的數(shù)據(jù)云端,每當(dāng)他們?cè)L問(wèn)網(wǎng)址就會(huì)有數(shù)據(jù)留下。
?
The thread that ties most of these applications together is that data collected from users provides added value. Whether that data is search terms, voice samples, or product reviews, the users are in a feedback loop in which they contribute to the products they use. That’s the beginning of data science.
這根把這些應(yīng)用的大多數(shù)連起來(lái)的線是從提供額外價(jià)值的用戶那里收集來(lái)的。數(shù)據(jù)是否是搜索條目,語(yǔ)音樣本,或產(chǎn)品評(píng)價(jià),用戶是在一個(gè)為他們使用的產(chǎn)品做貢獻(xiàn)的反饋循環(huán)中。那就是數(shù)據(jù)科學(xué)的開(kāi)始。
?
In the last few years, there has been an explosion in the amount of data that’s available. Whether we’re talking about web server logs, tweet streams, online transaction records, “citizen science,” data from sensors, government data, or some other source, the problem isn’t finding data, it’s figuring out what to do with it. And it’s not just companies using their own data, or the data contributed by their users. It’s increasingly common to mashup data from a number of sources. “Data Mashups in R” analyzes mortgage foreclosures in Philadelphia County by taking a public report from the county sheriff’s office, extracting addresses and using Yahoo to convert the addresses to latitude and longitude, then using the geographical data to place the foreclosures on a map (another data source), and group them by neighborhood, valuation, neighborhood per-capita income, and other socio-economic factors.
在過(guò)去的一些年里,可用數(shù)據(jù)的量級(jí)有了爆炸性的增長(zhǎng)。無(wú)論我們是否在討論網(wǎng)絡(luò)服務(wù)器日志,推特流,在線交易記錄,“公民科學(xué)”,來(lái)自傳感器、政府?dāng)?shù)據(jù)和其他來(lái)源的數(shù)據(jù),問(wèn)題不是在于尋找數(shù)據(jù),而是弄清楚該如何處理數(shù)據(jù)。公司不僅僅是使用他們自己的數(shù)據(jù),或由他們的用戶貢獻(xiàn)的數(shù)據(jù)?;旌喜煌瑏?lái)源的數(shù)據(jù)越來(lái)越普遍?!禗ata Mashups in R》通過(guò)獲取縣警長(zhǎng)辦公室的一份公共報(bào)告,分析了費(fèi)城縣的喪失抵押品贖回權(quán),提取地址并使用雅虎將地址轉(zhuǎn)化為經(jīng)度和維度,然后使用地理數(shù)據(jù)將抵押品贖回權(quán)的取消放入一張地圖上(地圖是另一個(gè)數(shù)據(jù)來(lái)源),并通過(guò)社區(qū)關(guān)系,估值,社區(qū)人均收入和其它社會(huì)經(jīng)濟(jì)因素來(lái)將其分組。
?
The question facing every company today, every startup, every non-profit, every project site that wants to attract a community, is how to use data effectively -- not just their own data, but all the data that’s available and relevant. Using data effectively requires something different from traditional statistics,
where actuaries in business suits perform arcane but fairly well-defined kinds of analysis. What differentiates data science from statistics is that data science is a holistic approach. We’re increasingly finding data in the wild, and data scientists are involved with gathering data, massaging it into a tractable form, making it tell its story, and presenting that story to others.
如何有效地使用數(shù)據(jù),這個(gè)問(wèn)題面向今天的每家公司,每個(gè)初創(chuàng)公司,每個(gè)非盈利公司,每個(gè)想要吸引社區(qū)的項(xiàng)目網(wǎng)站——不止是他們自己的數(shù)據(jù),而是所有有用和有關(guān)的數(shù)據(jù)。有效地使用數(shù)據(jù)需要一些與穿著商業(yè)套裝的精算師進(jìn)行的晦澀難懂但是定義明確的傳統(tǒng)統(tǒng)計(jì)學(xué)不一樣的東西。區(qū)分?jǐn)?shù)據(jù)科學(xué)與統(tǒng)計(jì)學(xué)的是,數(shù)據(jù)科學(xué)是一種全面整體的方式。我們?cè)絹?lái)越多地?zé)o序無(wú)意識(shí)地發(fā)現(xiàn)數(shù)據(jù),而數(shù)據(jù)科學(xué)家陷入收集數(shù)據(jù),包裝數(shù)據(jù)成為一種易處理的形式,讓數(shù)據(jù)說(shuō)出自己的故事,并數(shù)據(jù)的故事傳遞給其它人。
注:數(shù)據(jù)科學(xué)中數(shù)據(jù)的價(jià)值不明確,因?yàn)閿?shù)據(jù)是財(cái)富,不同的視角,也得出不同的結(jié)果,所以,數(shù)據(jù)科學(xué)肯定不像統(tǒng)計(jì)學(xué)一樣要求晦澀但是精確。in the wild在自然環(huán)境中,處于野生狀態(tài),所以翻譯成無(wú)序無(wú)意識(shí)地。
?
To get a sense for what skills are required, let’s look at the data lifecycle: where it comes from, how you use it, and where it goes.
要了解需要哪些技能,讓我們看看數(shù)據(jù)生命周期:它是從哪里來(lái),你要如何使用它,和它將去往哪里。
?